What Is Ordinal Data and Its Applications in Statistics
Table of contents
In statistics, ordinal data is one of the most vital concepts because it can put groups in a meaningful order. Such data is crucial for looking at frequency distributions, which count and study the number of data points that fall into certain categories to find patterns and trends. While nominal data, ordinal data, interval data, and ratio data are all types of data, ordinal data stands out because it can group and rank its subjects without saying how much they vary. Because of this, ordinal data is very useful in polls, statistical tests, and other situations where answers or observations can be ranked but not measured exactly.
The main points to be covered include:
- Understanding what ordinal data is and how it differs from other types of data.
- Illustrating ordinal data with real-world examples to clarify its practical uses.
- A detailed comparison with nominal data, interval, and ratio to highlight the unique features of each.
- An overview of statistical methods appropriate for displaying ordinal data, acknowledging their limitations in quantification.
In the next parts, we’ll talk about these topics in more detail, giving you a full picture of the role and methods of ordinal data in analyzing ordinal data. In addition, this exploration will also teach us how to use these ideas effectively.
Ordinal Data vs. Other Data Types
Knowing the differences between the four main types of data — nominal, ordinal, interval, and ratio — is essential when choosing the right data analysis methods. This kind of measurement offers different levels of accuracy and difficulty.
Nominal data, the most basic form, categorizes without implying any order among the categories. It’s used for labeled data or naming values, such as marital status or eye color, without a hierarchical structure. In contrast, ordinal data introduces a level of order or ranking among its categories, like economic status categorized as ‘wealthy’, ‘middle income’, or ‘poor’, yet it lacks defined intervals between these rankings.
When we look at interval and ratio data, which are both numbers and give us more information because they are more complicated, the difference becomes clearer. Interval data, while classifying and ranking like ordinal data, also specifies the intervals between categories, such as temperature measurements in Celsius or Fahrenheit. However, it lacks a true zero, meaning zero does not denote the absence of the quantity measured. Ratio data, on the other hand, builds upon interval by including a true zero, offering the most precise level of measurement. This makes it possible to say that when a variable equals zero, none of it exists, as exemplified by height measurements.
Nevertheless, categorical data like bar graphs can help researchers communicate complex relationships and data types. They bridge the gap between statistical analysis and practical comprehension by making easy to grasp and analyze nominal and ordinal data.
Data Type | Classification | Order | Equal Intervals | True Zero | Examples |
Nominal Data | Yes | No | No | No | Marital status, hair color, type of car |
Ordinal Data | Yes | Yes | No | No | Economic status (wealthy, middle income, poor), satisfaction rating (satisfied, neutral, dissatisfied) |
Interval Data | Yes | Yes | Yes | No | Temperature in Celsius or Fahrenheit, IQ scores, dates on the calendar |
Ratio Data | Yes | Yes | Yes | Yes | Height, weight, age, annual income |
Understanding these differences is significant in data analysis for several reasons:
- Choice of Statistical Techniques: The type of data you’re working with directly influences the statistical methods you can employ. For instance, certain statistical tests work by testing hypotheses and drawing conclusions based on knowledge, and they are only appropriate for nominal data or ordinal data, while others require the precision of ratio or interval data.
- Interpretation Accuracy: The level of measurement affects how accurately you can interpret data. Ordinal and nominal data provide basic insights but with limited precision. In contrast, ratio and interval data allow for more detailed and quantifiable analysis.
- Research Design: Early identification of the appropriate data type can guide the design of research studies.
By the way, you might want to look into more tools and guides, like how to do a case study analysis, to learn more about how these types of data affect the analysis process and how to use each one effectively in your research. And you can read more about this in our post.
Distinguish Ordinal Data From Other Data Types
Distinguishing ordinal data from other data types is crucial for proper analysis in both everyday life and research. Ordinal data is unique in that it provides a ranked order among more or two values without specifying the exact distance between them. For example, in everyday life, product ratings (such as 1 to 5 stars) or levels of education (high school, bachelor’s, master’s, PhD) are ordinal, as they indicate a sequence without revealing the precise gap between ranks. In research, stages of disease (early, middle, late) or socio-economic status (low, middle, high) serve as ordinal data, allowing for categorization and prioritization based on severity or wealth, respectively.
Importance of Ordinal Data in Statistics
Ordinal data’s utility in statistical analysis is underscored by its ability to rank entities, which is crucial for drawing conclusions based on attributes or responses. This data type is particularly valuable for understanding trends, preferences, and attitudes, offering insights without the need for precise measurement intervals. For example, in assessing income level, ordinal data can classify respondents into low, medium, and high categories, facilitating analysis of consumer behavior or social trends across different socioeconomic groups.
In research involving repeated measures, ordinal data allows for the examination of changes over time within the same subjects. Similarly, when comparing two independent samples, ordinal data enables analysts to discern patterns and differences in rankings that might indicate significant variations in preferences, attitudes, or experiences between groups.
The information you get from looking at ordinal variables can help you make decisions, come up with policies, and come up with strategies in many different areas. By knowing how responses are ordered, researchers and analysts can find patterns and trends that can help them learn about things like health outcomes, public opinion, and consumer behaviour.
If you want to learn more about ordinal variables and improve your statistical analysis skills, PapersOwl.com has a lot of tools and guides with categorical data that can help you with everything from basic ideas to more advanced analytical techniques.
Collecting Ordinal Data
Collecting ordinal data is integral to research, particularly when outcomes are best represented through ranked orders or scales. Commonly used methods like surveys, questionnaires, and observations are tailored to capture this type of data efficiently. The design of effective tools for gathering ordinal data, such as the Likert scale and ranking questions, hinges on a clear alignment with the research objectives and the nuances of the data’s intended analysis. These tools are adept at providing respondents with a structured format to express their preferences or opinions along a defined continuum.
For instance, in designing a questionnaire to assess income level, questions might categorize income ranges or ask respondents to rank their income level relative to societal norms. When examining the effectiveness of a new educational program, researchers might employ the Wilcoxon matched pairs test to analyze pre- and post-test scores of participants, where these scores are ranked ordinally to observe improvements.
A survey might include a Likert scale asking participants to express their agreement with statements about environmental conservation, from “Strongly Disagree” to “Strongly Agree.” This method captures the intensity of participants’ attitudes, providing insights that are invaluable for policy development or educational initiatives. Similarly, Mood’s median test could be used to compare responses across two independent samples, such as comparing attitudes towards renewable energy between urban and rural residents, highlighting the median value as a measure of central tendency.
Ordinal data’s application extends to everyday contexts, such as consumer feedback where customers rank their dining experience from “Poor” to “Excellent.” This feedback helps restaurants identify areas for improvement and understand customer preferences. In healthcare, patient satisfaction surveys often use ordinal scales to rate various aspects of care, from “Unsatisfied” to “Very Satisfied,” guiding quality improvement efforts. By the way, understanding core ideas like hypothesis formation can help explain the systematic approach to data gathering and analysis in research.
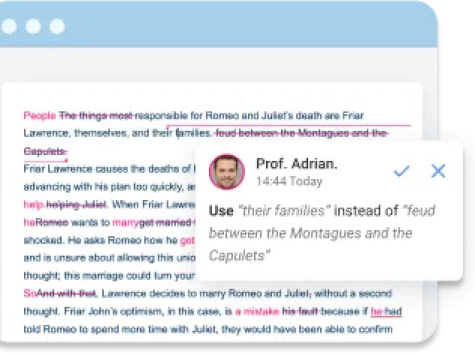
Analyzing Ordinal Data
In the field of data analytics, analyzing ordinal scale requires a blend of inferential and descriptive statistics. This approach helps to interpret the data collected, focusing on the rank order of responses without assuming equal distances between them. Understanding how to navigate through the complexities of ordinal data, for example, with tests, such as the Mann-Whitney U test or the Wilcoxon rank sum test, is crucial for effective data collection and subsequent statistical studies.
Descriptive Statistics for Ordinal Data
When dealing with ordinal data, descriptive statistics provide a preliminary overview. Key components include:
- Frequency distribution to display the count or percentage of each response.
- The mode and median as measures of central value, offering insights into the most common and middle responses, respectively.
- The range to show the spread of responses, giving a sense of variability within the data.
Example
Imagine surveying to evaluate public opinion on a new urban policy. Respondents are asked to rank their support on a scale from “Strongly oppose” to “Strongly support.”
A frequency distribution might reveal the following:
- Strongly oppose: 15%
- Oppose: 20%
- Neutral: 30%
- Support: 25%
- Strongly support: 10%
To depict these results visually, a bar chart could be created, placing support levels on the x-axis and percentage of responses on the y-axis, accurately reflecting the ordinal data’s structured order.
Central Value:
- The mode of the survey results is “Neutral,” indicating it’s the most frequent response.
- If calculating the median in this distribution, and given an even number of responses, we might find “Neutral” and “Support” as the middle of two values. Since two ordinal variables do not allow for precise mean calculation due to the lack of known intervals between ranks, we highlight the median as a key central value to represent the dataset’s midpoint.
Inferential Statistics for Ordinal Data
For deeper analysis, inferential statistics come into play, utilizing non-parametric tests tailored for ordinal data. These tests are designed to draw conclusions about the population based on sample data, without assuming a normal distribution.
Non-parametric tests suitable for ordinal data include:
- Mood’s median test to compare median responses across different groups.
- Wilcoxon matched-pairs signed-rank test for paired samples to determine if there are significant differences in their rankings.
- Mann-Whitney U test for comparing two separate samples.
- Spearman’s rank correlation coefficient to assess the relationship between two sets of ordinal data.
Example
Suppose we want to compare the level of support for the urban policy between two neighborhoods. Using Mood’s median test, we could determine if there is a significant difference in median levels of support, thus applying inferential statistics to draw meaningful conclusions from our data collection efforts.
In data analytics, these statistical methods are indispensable to analyze ordinal data, as they respect the data’s ordinal nature while allowing researchers to draw conclusions based on observed trends and differences. This analytical process is fundamental to transforming ordinal variables into actionable insights, highlighting the importance of choosing the right statistical techniques in the realm of statistical analyses.
Applications of Ordinal Data
Ordinal data, with its ability to rank order without specifying a clearly defined interval between rankings, finds versatile applications across numerous fields, including education, marketing, and social sciences. This type of data is particularly valuable for capturing quantitative variables that are categorized based on their relative position or ranking.
In education, ordinal data is used to assess student satisfaction or learning outcomes through surveys that ask respondents to rate their experience or understanding from “Very Poor” to “Excellent.” Such data helps educators identify areas of strength and opportunities for improvement. They use summary statistics and measures of central tendency to get a snapshot of the collective student body’s sentiment or comprehension levels. For those tackling a statistics assignment on such topics, resources like PapersOwl can provide invaluable assistance in analyzing and interpreting these types of data.
Marketing professionals leverage ordinal data to gauge consumer preferences and product rankings. For example, a company may use customer feedback forms that include ordinal scales to rate product features or overall satisfaction. Analyzing these responses provides summary statistics that highlight the most and least favored aspects of a product, guiding future product development and marketing strategies.
In the realm of social sciences, ordinal variables aid in understanding societal trends and behaviors. Surveys measuring attitudes towards social issues, such as economic policy or public health measures, often employ ordinal scales. Researchers then apply measures of central tendency to summarize public opinion, offering valuable insights into the population’s stance on various matters.
These applications underscore the importance of ordinal data in drawing meaningful conclusions from rankings, without the need for a clearly defined interval between each rank.
Limitations of Ordinal Data
The utilization of ordinal variables in research, while beneficial for capturing rankings and preferences, comes with its set of limitations, especially when it comes to conducting certain statistical analyses. One of the main constraints is its inability to quantify the exact difference between ranked positions, unlike ratio or ratio data. This limitation can significantly impact the depth of analysis and the precision of research outcomes and interpretations.
For instance, when collecting ordinal data, researchers can rank ordinal data examples like levels of education or satisfaction. However, they cannot ascertain the precise magnitude of difference between these ranks, which is feasible with numerical data types. This makes it challenging to apply statistical methods that rely on arithmetic operations, such as calculating the mean or standard deviation, limiting the analytical techniques to non-parametric tests and measures of central tendency like the median or mode.
Furthermore, ordinal data’s limitation in detailing the relationship between ranked categories means that nominal variables and independent variable data samples might be analyzed with more accuracy using other data types. This is because examples of ordinal variables do not permit the use of parametric tests, which require assumptions about the distribution of the data, potentially leading to less statistically powerful insights.
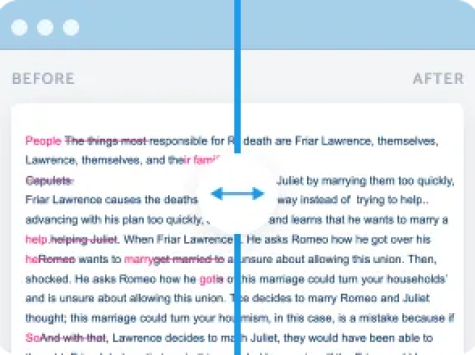
Unraveling the Nuances of Ordinal Data
By bridging the gap between simple classification and the quantification of quantitative factors, ordinal data is an important part of statistical analysis. Through this post, we’ve taken a look at what is ordinal in statistics, distinguishing it from other data types by its unique capability to rank order entities based on their characteristics or responses.
The key takeaways from our discussion include:
- Ordinal data’s fundamental role is to offer a method to classify and prioritize quantitative variables based on a relative scale, enhancing the analysis of frequency distributions and enabling researchers to identify patterns and trends.
- The importance of selecting appropriate statistical methods that align with ordinal data’s properties, such as non-parametric tests, Likert scale, and measures of central tendency like the median and mode, which serve as crucial summary statistics.
- The practical applications of ordinal variables across various fields, from education and marketing to social sciences, where it facilitate the understanding of trends, preferences, and attitudes, thereby informing decision-making and policy formulation.
However, it’s also crucial to acknowledge the limitations associated with ordinal data, particularly its constraints in conducting certain statistical studies due to the lack of a clearly defined interval. These limitations underscore the necessity for careful consideration in the choice of analytical techniques and the interpretation of research outcomes.